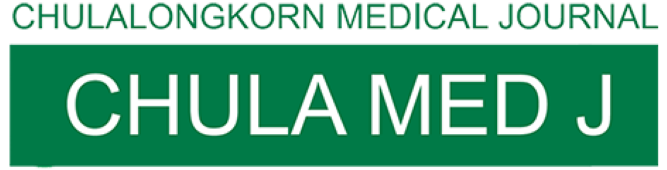
Abstract
Background: In Thailand, the high prevalence of depression, particularly in rural areas with limited mental health services, poses significant challenges. Advanced technologies like digital phenotyping and artificial intelligence (AI), particularly Natural Language Processing (NLP) and Large Language Models (LLMs), offer promising solutions by analyzing digital data to detect early signs of depression.
Objective: This study evaluated the performance of the “Detection and Monitoring Intelligence Network of Depression (DMIND)” application, an AI-powered screening tool for detecting depression in Thai adults that analyzes behavioral data from participant responses using machine learning algorithms, such as NLP models and LLMs. The study aimed to determine the agreement of the DMIND AI model and the Thai version of the Hamilton Depression Rating Scale (HDRS-17 Thai) in classifying depression severity.
Methods: This cross-sectional study recruited 388 participants from one tertiary care hospital and two psychiatric hospitals. Initially, participants used the DMIND application, where they were asked to answer a series of questions. Their response in the application was recorded and a pre-trained AI model predicted their depression severity. Subsequently, a trained nurse or psychologist then assessed participants using the HDRS-17 Thai to establish a baseline measure of depression severity.
Statistical analysis involved comparing the depression severity classifications from the DMIND AI model with the HDRS-17 Thai. Cohen’s kappa coefficient, sensitivity, specificity, and predictive values were used to evaluate the agreement between the two assessments.
Results: Our DMIND application demonstrated moderate agreement with the HDRS-17 Thai, indicating substantial consistency in depression severity classification. The tool showed high sensitivity (87.30%) and moderate specificity (59.50%), with strong negative predictive values for detecting depression.
Conclusion: The AI-powered DMIND application effectively screens for depression by analyzing digital data from participants’ responses. Its moderate agreement with a traditional clinical assessment and strong diagnostic performance highlights its potential as a scalable, accessible tool for mental health management in Thailand. Integrating AI tools like the DMIND into the public health infrastructure could significantly enhance the accessibility, accuracy, and responsiveness of mental health services, particularly in underserved regions, potentially revolutionizing the management and treatment of depression across the country
DOI
10.56808/2673-060X.5495
First Page
1
Last Page
28
Recommended Citation
Hemrungrojn, Solaphat; Saengsai, Kittipoch; Jakkrawankul, Pasit; Kiattiporn-Opas, Chanyanart; Chaichareenon, Kantapat; Amrapala, Arisara; Lapanan, Kulvara; Achakulvisut, Titipat; Vateekul, Peerapol; Nupairoj, Natawut; Phutrakool, Phanupong; Yodlorchai, Rapinpat; Hiransuthikul, Narin; and Hengprapom, Sarunya
(2024)
"Evaluating the Accuracy and Concordance of the DMIND AI Application with A Renowned Depression Assessment Tool in Thai Adults,"
Chulalongkorn Medical Journal: Vol. 68:
Iss.
4, Article 4.
DOI: https://doi.org/10.56808/2673-060X.5495
Available at:
https://digital.car.chula.ac.th/clmjournal/vol68/iss4/4